14.2: Affine Transforms
- Last updated
- Sep 17, 2022
- Save as PDF
- Page ID
- 65563
( \newcommand{\kernel}{\mathrm{null}\,}\)
In this section, we are going to explore different types of transformation matrices. The following code is designed to demonstrate the properties of some different transformation matrices.
Do This
Review the following code.
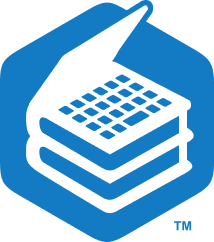
Login with LibreOne to run this code cell interactively.
If you have already signed in, please refresh the page.
#Some python packages we will be using %matplotlib inline import numpy as np import matplotlib.pylab as plt from mpl_toolkits.mplot3d import Axes3D #Lets us make 3D plots import numpy as np import sympy as sym sym.init_printing(use_unicode=True) # Trick to make matrixes look nice in jupyter
#Some python packages we will be using %matplotlib inline import numpy as np import matplotlib.pylab as plt from mpl_toolkits.mplot3d import Axes3D #Lets us make 3D plots import numpy as np import sympy as sym sym.init_printing(use_unicode=True) # Trick to make matrixes look nice in jupyter
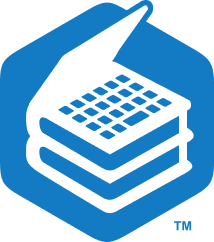
Login with LibreOne to run this code cell interactively.
If you have already signed in, please refresh the page.
# Define some points x = [0.0, 0.0, 2.0, 8.0, 10.0, 10.0, 8.0, 4.0, 3.0, 3.0, 4.0, 6.0, 7.0, 7.0, 10.0, 10.0, 8.0, 2.0, 0.0, 0.0, 2.0, 6.0, 7.0, 7.0, 6.0, 4.0, 3.0, 3.0, 0.0] y = [0.0, -2.0, -4.0, -4.0, -2.0, 2.0, 4.0, 4.0, 5.0, 7.0, 8.0, 8.0, 7.0, 6.0, 6.0, 8.0, 10.0, 10.0, 8.0, 4.0, 2.0, 2.0, 1.0, -1.0, -2.0, -2.0, -1.0, 0.0, 0.0] con = [ 1.0 for i in range(len(x))] p = np.matrix([x,y,con]) mp = p.copy() #Plot Points plt.plot(mp[0,:].tolist()[0],mp[1,:].tolist()[0], color='green'); plt.axis('scaled'); plt.axis([-10,20,-15,15]); plt.title('Start Location');
# Define some points x = [0.0, 0.0, 2.0, 8.0, 10.0, 10.0, 8.0, 4.0, 3.0, 3.0, 4.0, 6.0, 7.0, 7.0, 10.0, 10.0, 8.0, 2.0, 0.0, 0.0, 2.0, 6.0, 7.0, 7.0, 6.0, 4.0, 3.0, 3.0, 0.0] y = [0.0, -2.0, -4.0, -4.0, -2.0, 2.0, 4.0, 4.0, 5.0, 7.0, 8.0, 8.0, 7.0, 6.0, 6.0, 8.0, 10.0, 10.0, 8.0, 4.0, 2.0, 2.0, 1.0, -1.0, -2.0, -2.0, -1.0, 0.0, 0.0] con = [ 1.0 for i in range(len(x))] p = np.matrix([x,y,con]) mp = p.copy() #Plot Points plt.plot(mp[0,:].tolist()[0],mp[1,:].tolist()[0], color='green'); plt.axis('scaled'); plt.axis([-10,20,-15,15]); plt.title('Start Location');
Example Scaling Matrix
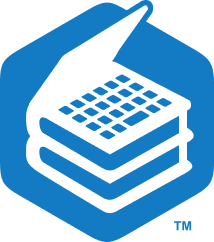
Login with LibreOne to run this code cell interactively.
If you have already signed in, please refresh the page.
#Example Scaling Matrix #Define Matrix scale = 0.5 #The amount that coordinates are scaled. S = np.matrix([[scale,0,0], [0,scale,0], [0,0,1]]) #Apply matrix mp = p.copy() mp = S*mp #Plot points after transform plt.plot(mp[0,:].tolist()[0],mp[1,:].tolist()[0], color='green') plt.axis('scaled') plt.axis([-10,20,-15,15]) plt.title('After Scaling') #Uncomment the next line if you want to see the original. # plt.plot(p[0,:].tolist()[0],p[1,:].tolist()[0], color='blue',alpha=0.3); sym.Matrix(S)
#Example Scaling Matrix #Define Matrix scale = 0.5 #The amount that coordinates are scaled. S = np.matrix([[scale,0,0], [0,scale,0], [0,0,1]]) #Apply matrix mp = p.copy() mp = S*mp #Plot points after transform plt.plot(mp[0,:].tolist()[0],mp[1,:].tolist()[0], color='green') plt.axis('scaled') plt.axis([-10,20,-15,15]) plt.title('After Scaling') #Uncomment the next line if you want to see the original. # plt.plot(p[0,:].tolist()[0],p[1,:].tolist()[0], color='blue',alpha=0.3); sym.Matrix(S)
Example Translation Matrix
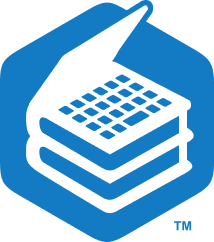
Login with LibreOne to run this code cell interactively.
If you have already signed in, please refresh the page.
#Example Translation Matrix #Define Matrix dx = 1 #The amount shifted in the x-direction dy = 1 #The amount shifted in the y-direction T = np.matrix([[1,0,dx], [0,1,dy], [0,0,1]]) #Apply matrix mp = p.copy() mp = T*mp #Plot points after transform plt.plot(mp[0,:].tolist()[0],mp[1,:].tolist()[0], color='green') plt.axis('scaled') plt.axis([-10,20,-15,15]) plt.title('After Translation') #Uncomment the next line if you want to see the original. # plt.plot(p[0,:].tolist()[0],p[1,:].tolist()[0], color='blue',alpha=0.3); sym.Matrix(T)
#Example Translation Matrix #Define Matrix dx = 1 #The amount shifted in the x-direction dy = 1 #The amount shifted in the y-direction T = np.matrix([[1,0,dx], [0,1,dy], [0,0,1]]) #Apply matrix mp = p.copy() mp = T*mp #Plot points after transform plt.plot(mp[0,:].tolist()[0],mp[1,:].tolist()[0], color='green') plt.axis('scaled') plt.axis([-10,20,-15,15]) plt.title('After Translation') #Uncomment the next line if you want to see the original. # plt.plot(p[0,:].tolist()[0],p[1,:].tolist()[0], color='blue',alpha=0.3); sym.Matrix(T)
Example Reflection Matrix
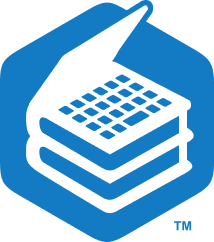
Login with LibreOne to run this code cell interactively.
If you have already signed in, please refresh the page.
#Example Reflection Matrix #Define Matrix Re = np.matrix([[1,0,0],[0,-1,0],[0,0,1]]) ## Makes all y-values opposite so it reflects over the x-axis. #Apply matrix mp = p.copy() mp = Re*mp #Plot points after transform plt.plot(mp[0,:].tolist()[0],mp[1,:].tolist()[0], color='green') plt.axis('scaled') plt.axis([-10,20,-15,15]) #Uncomment the next line if you want to see the original. # plt.plot(p[0,:].tolist()[0],p[1,:].tolist()[0], color='blue',alpha=0.3); sym.Matrix(Re)
#Example Reflection Matrix #Define Matrix Re = np.matrix([[1,0,0],[0,-1,0],[0,0,1]]) ## Makes all y-values opposite so it reflects over the x-axis. #Apply matrix mp = p.copy() mp = Re*mp #Plot points after transform plt.plot(mp[0,:].tolist()[0],mp[1,:].tolist()[0], color='green') plt.axis('scaled') plt.axis([-10,20,-15,15]) #Uncomment the next line if you want to see the original. # plt.plot(p[0,:].tolist()[0],p[1,:].tolist()[0], color='blue',alpha=0.3); sym.Matrix(Re)
Example Rotation Matrix
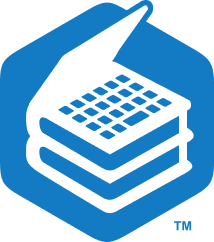
Login with LibreOne to run this code cell interactively.
If you have already signed in, please refresh the page.
#Example Rotation Matrix #Define Matrix degrees = 30 theta = degrees * np.pi / 180 ##Make sure to always convert from degrees to radians. # Rotates the points 30 degrees counterclockwise. R = np.matrix([[np.cos(theta),-np.sin(theta),0],[np.sin(theta), np.cos(theta),0],[0,0,1]]) #Apply matrix mp = p.copy() mp = R*mp #Plot points after transform plt.plot(mp[0,:].tolist()[0],mp[1,:].tolist()[0], color='green') plt.axis('scaled') plt.axis([-10,20,-15,15]) #Uncomment the next line if you want to see the original. # plt.plot(p[0,:].tolist()[0],p[1,:].tolist()[0], color='blue',alpha=0.3); sym.Matrix(R)
#Example Rotation Matrix #Define Matrix degrees = 30 theta = degrees * np.pi / 180 ##Make sure to always convert from degrees to radians. # Rotates the points 30 degrees counterclockwise. R = np.matrix([[np.cos(theta),-np.sin(theta),0],[np.sin(theta), np.cos(theta),0],[0,0,1]]) #Apply matrix mp = p.copy() mp = R*mp #Plot points after transform plt.plot(mp[0,:].tolist()[0],mp[1,:].tolist()[0], color='green') plt.axis('scaled') plt.axis([-10,20,-15,15]) #Uncomment the next line if you want to see the original. # plt.plot(p[0,:].tolist()[0],p[1,:].tolist()[0], color='blue',alpha=0.3); sym.Matrix(R)
Example Shear Matrix
Combine Transforms
We have five transforms R, S, T, Re, and SH
Do This
Construct a (3×3) transformation Matrix (called M) which combines these five transforms into a single matrix. You can choose different orders for these five matrix, then compare your result with other students.
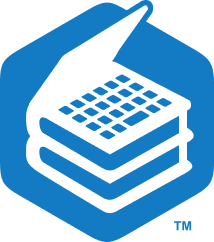
Login with LibreOne to run this code cell interactively.
If you have already signed in, please refresh the page.
#Put your code here
#Put your code here
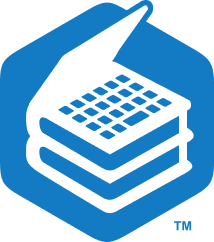
Login with LibreOne to run this code cell interactively.
If you have already signed in, please refresh the page.
#Plot combined transformed points mp = p.copy() mp = M*mp plt.plot(mp[0,:].tolist()[0],mp[1,:].tolist()[0], color='green'); plt.axis('scaled'); plt.axis([-10,20,-15,15]); plt.title('Start Location');
#Plot combined transformed points mp = p.copy() mp = M*mp plt.plot(mp[0,:].tolist()[0],mp[1,:].tolist()[0], color='green'); plt.axis('scaled'); plt.axis([-10,20,-15,15]); plt.title('Start Location');
Questions
Did you can get the same result with others? You can compare the matrix M to see the difference. If not, can you explain why it happens?
Interactive Example
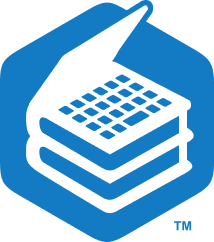
Login with LibreOne to run this code cell interactively.
If you have already signed in, please refresh the page.
from ipywidgets import interact,interact_manual def affine_image(angle=0,scale=1.0,dx=0,dy=0, shx=0, shy=0): theta = -angle/180 * np.pi plt.plot(p[0,:].tolist()[0],p[1,:].tolist()[0], color='green') S = np.matrix([[scale,0,0], [0,scale,0], [0,0,1]]) SH = np.matrix([[1,shx,0], [shy,1,0], [0,0,1]]) T = np.matrix([[1,0,dx], [0,1,dy], [0,0,1]]) R = np.matrix([[np.cos(theta),-np.sin(theta),0],[np.sin(theta), np.cos(theta),0],[0,0,1]]) #Full Transform FT = T*SH*R*S; #Apply Transforms p2 = FT*p; #Plot Output plt.plot(p2[0,:].tolist()[0],p2[1,:].tolist()[0], color='black') plt.axis('scaled') plt.axis([-10,20,-15,15]) return sym.Matrix(FT)
from ipywidgets import interact,interact_manual def affine_image(angle=0,scale=1.0,dx=0,dy=0, shx=0, shy=0): theta = -angle/180 * np.pi plt.plot(p[0,:].tolist()[0],p[1,:].tolist()[0], color='green') S = np.matrix([[scale,0,0], [0,scale,0], [0,0,1]]) SH = np.matrix([[1,shx,0], [shy,1,0], [0,0,1]]) T = np.matrix([[1,0,dx], [0,1,dy], [0,0,1]]) R = np.matrix([[np.cos(theta),-np.sin(theta),0],[np.sin(theta), np.cos(theta),0],[0,0,1]]) #Full Transform FT = T*SH*R*S; #Apply Transforms p2 = FT*p; #Plot Output plt.plot(p2[0,:].tolist()[0],p2[1,:].tolist()[0], color='black') plt.axis('scaled') plt.axis([-10,20,-15,15]) return sym.Matrix(FT)
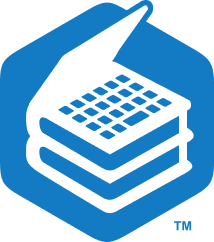
Login with LibreOne to run this code cell interactively.
If you have already signed in, please refresh the page.
##TODO: Modify this line of code interact(affine_image, angle=(-180,180), scale_manual=(0.01,2), dx=(-5,15,0.5), dy=(-15,15,0.5), shx = (-1,1,0.1), shy = (-1,1,0.1));
##TODO: Modify this line of code interact(affine_image, angle=(-180,180), scale_manual=(0.01,2), dx=(-5,15,0.5), dy=(-15,15,0.5), shx = (-1,1,0.1), shy = (-1,1,0.1));
The following command can also be used but it may be slow on some peoples computers.
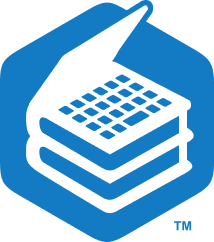
Login with LibreOne to run this code cell interactively.
If you have already signed in, please refresh the page.
##TODO: Modify this line of code #interact(affine_image, angle=(-180,180), scale=(0.01,2), # dx=(-5,15,0.5), dy=(-15,15,0.5), shx = (-1,1,0.1), shy = (-1,1,0.1));
##TODO: Modify this line of code #interact(affine_image, angle=(-180,180), scale=(0.01,2), # dx=(-5,15,0.5), dy=(-15,15,0.5), shx = (-1,1,0.1), shy = (-1,1,0.1));
Do This
Using the above interactive enviornment to see if you can figure out the transformation matrix to make the following image:
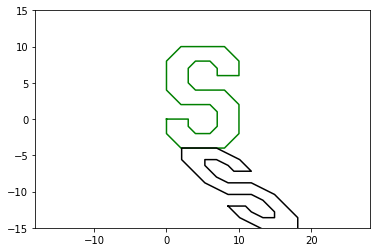
Question
What where the input values?