1.2: Sampling Designs
- Page ID
- 105809
\( \newcommand{\vecs}[1]{\overset { \scriptstyle \rightharpoonup} {\mathbf{#1}} } \)
\( \newcommand{\vecd}[1]{\overset{-\!-\!\rightharpoonup}{\vphantom{a}\smash {#1}}} \)
\( \newcommand{\id}{\mathrm{id}}\) \( \newcommand{\Span}{\mathrm{span}}\)
( \newcommand{\kernel}{\mathrm{null}\,}\) \( \newcommand{\range}{\mathrm{range}\,}\)
\( \newcommand{\RealPart}{\mathrm{Re}}\) \( \newcommand{\ImaginaryPart}{\mathrm{Im}}\)
\( \newcommand{\Argument}{\mathrm{Arg}}\) \( \newcommand{\norm}[1]{\| #1 \|}\)
\( \newcommand{\inner}[2]{\langle #1, #2 \rangle}\)
\( \newcommand{\Span}{\mathrm{span}}\)
\( \newcommand{\id}{\mathrm{id}}\)
\( \newcommand{\Span}{\mathrm{span}}\)
\( \newcommand{\kernel}{\mathrm{null}\,}\)
\( \newcommand{\range}{\mathrm{range}\,}\)
\( \newcommand{\RealPart}{\mathrm{Re}}\)
\( \newcommand{\ImaginaryPart}{\mathrm{Im}}\)
\( \newcommand{\Argument}{\mathrm{Arg}}\)
\( \newcommand{\norm}[1]{\| #1 \|}\)
\( \newcommand{\inner}[2]{\langle #1, #2 \rangle}\)
\( \newcommand{\Span}{\mathrm{span}}\) \( \newcommand{\AA}{\unicode[.8,0]{x212B}}\)
\( \newcommand{\vectorA}[1]{\vec{#1}} % arrow\)
\( \newcommand{\vectorAt}[1]{\vec{\text{#1}}} % arrow\)
\( \newcommand{\vectorB}[1]{\overset { \scriptstyle \rightharpoonup} {\mathbf{#1}} } \)
\( \newcommand{\vectorC}[1]{\textbf{#1}} \)
\( \newcommand{\vectorD}[1]{\overrightarrow{#1}} \)
\( \newcommand{\vectorDt}[1]{\overrightarrow{\text{#1}}} \)
\( \newcommand{\vectE}[1]{\overset{-\!-\!\rightharpoonup}{\vphantom{a}\smash{\mathbf {#1}}}} \)
\( \newcommand{\vecs}[1]{\overset { \scriptstyle \rightharpoonup} {\mathbf{#1}} } \)
\( \newcommand{\vecd}[1]{\overset{-\!-\!\rightharpoonup}{\vphantom{a}\smash {#1}}} \)
\(\newcommand{\avec}{\mathbf a}\) \(\newcommand{\bvec}{\mathbf b}\) \(\newcommand{\cvec}{\mathbf c}\) \(\newcommand{\dvec}{\mathbf d}\) \(\newcommand{\dtil}{\widetilde{\mathbf d}}\) \(\newcommand{\evec}{\mathbf e}\) \(\newcommand{\fvec}{\mathbf f}\) \(\newcommand{\nvec}{\mathbf n}\) \(\newcommand{\pvec}{\mathbf p}\) \(\newcommand{\qvec}{\mathbf q}\) \(\newcommand{\svec}{\mathbf s}\) \(\newcommand{\tvec}{\mathbf t}\) \(\newcommand{\uvec}{\mathbf u}\) \(\newcommand{\vvec}{\mathbf v}\) \(\newcommand{\wvec}{\mathbf w}\) \(\newcommand{\xvec}{\mathbf x}\) \(\newcommand{\yvec}{\mathbf y}\) \(\newcommand{\zvec}{\mathbf z}\) \(\newcommand{\rvec}{\mathbf r}\) \(\newcommand{\mvec}{\mathbf m}\) \(\newcommand{\zerovec}{\mathbf 0}\) \(\newcommand{\onevec}{\mathbf 1}\) \(\newcommand{\real}{\mathbb R}\) \(\newcommand{\twovec}[2]{\left[\begin{array}{r}#1 \\ #2 \end{array}\right]}\) \(\newcommand{\ctwovec}[2]{\left[\begin{array}{c}#1 \\ #2 \end{array}\right]}\) \(\newcommand{\threevec}[3]{\left[\begin{array}{r}#1 \\ #2 \\ #3 \end{array}\right]}\) \(\newcommand{\cthreevec}[3]{\left[\begin{array}{c}#1 \\ #2 \\ #3 \end{array}\right]}\) \(\newcommand{\fourvec}[4]{\left[\begin{array}{r}#1 \\ #2 \\ #3 \\ #4 \end{array}\right]}\) \(\newcommand{\cfourvec}[4]{\left[\begin{array}{c}#1 \\ #2 \\ #3 \\ #4 \end{array}\right]}\) \(\newcommand{\fivevec}[5]{\left[\begin{array}{r}#1 \\ #2 \\ #3 \\ #4 \\ #5 \\ \end{array}\right]}\) \(\newcommand{\cfivevec}[5]{\left[\begin{array}{c}#1 \\ #2 \\ #3 \\ #4 \\ #5 \\ \end{array}\right]}\) \(\newcommand{\mattwo}[4]{\left[\begin{array}{rr}#1 \amp #2 \\ #3 \amp #4 \\ \end{array}\right]}\) \(\newcommand{\laspan}[1]{\text{Span}\{#1\}}\) \(\newcommand{\bcal}{\cal B}\) \(\newcommand{\ccal}{\cal C}\) \(\newcommand{\scal}{\cal S}\) \(\newcommand{\wcal}{\cal W}\) \(\newcommand{\ecal}{\cal E}\) \(\newcommand{\coords}[2]{\left\{#1\right\}_{#2}}\) \(\newcommand{\gray}[1]{\color{gray}{#1}}\) \(\newcommand{\lgray}[1]{\color{lightgray}{#1}}\) \(\newcommand{\rank}{\operatorname{rank}}\) \(\newcommand{\row}{\text{Row}}\) \(\newcommand{\col}{\text{Col}}\) \(\renewcommand{\row}{\text{Row}}\) \(\newcommand{\nul}{\text{Nul}}\) \(\newcommand{\var}{\text{Var}}\) \(\newcommand{\corr}{\text{corr}}\) \(\newcommand{\len}[1]{\left|#1\right|}\) \(\newcommand{\bbar}{\overline{\bvec}}\) \(\newcommand{\bhat}{\widehat{\bvec}}\) \(\newcommand{\bperp}{\bvec^\perp}\) \(\newcommand{\xhat}{\widehat{\xvec}}\) \(\newcommand{\vhat}{\widehat{\vvec}}\) \(\newcommand{\uhat}{\widehat{\uvec}}\) \(\newcommand{\what}{\widehat{\wvec}}\) \(\newcommand{\Sighat}{\widehat{\Sigma}}\) \(\newcommand{\lt}{<}\) \(\newcommand{\gt}{>}\) \(\newcommand{\amp}{&}\) \(\definecolor{fillinmathshade}{gray}{0.9}\)Section 1: Sampling Designs and Biases
As previously stated, the two methods other than a census for obtaining information are sampling and experimentation. As we are already aware that the sampling error cannot be avoided, we should try to eliminate or minimize the non-sampling error. Next, we will discuss the sampling designs and different biases. One example of a non-sampling error is a sampling bias caused by the sampling method. In plain language, sampling bias is a measure of how "not representative" is the sample. One way to minimize the bias is to use a sampling procedure for which each possible sample of a given size is equally likely to be the one obtained. Such a procedure is called simple random sampling. The sample obtained from using such a procedure is called a simple random sample. So how exactly does one obtain a simple random sample? Let's pick a random sample of size 5! One way to arrange that is to have everyone write their names on a piece of paper and put it in a hat, and then randomly draw 5 pieces of paper from the hat.

We can classify the sampling based on whether the used piece of paper goes back in the hat or not. One is a sampling with replacement, whereby a member of the population can be selected more than once; the other is sampling without replacement, whereby a member of the population can be selected at most once. Unless we specify otherwise, a simple random sampling is done without replacement. Technically it is harder to perform the sampling without replacement as it requires additional effort of tracking and making sure the choices do not repeat. However, when a sample size is less than 5% of the population size the difference in results is almost negligible. But when population is small, or the sample size is large in relation to the size of the population there could be instances when with or without replacement matters.
Let’s decide whether a sampling with or without replacement is more appropriate in the following scenario?
- A teacher picks a random sample of 10 students to answer 10 questions throughout the duration of the class. I’d say that sampling with replacement is more appropriate in this case because as a teacher you don’t want a student think that he or she can’t be picked again after he was already picked once.
- A teacher picks a random sample of 10 students to attend the conference with him or her.
I’d say that sampling without replacement is definitely more appropriate in this case because a teacher can’t take the same student more than once on the same trip.
Obtaining a simple random sample by picking slips of paper out of a box is usually impractical, especially when the population is large. Fortunately, we can use several practical procedures to get simple random samples. One common method involves a table of random numbers, however, nowadays computers can easily replace the table.
A simple random sampling is the most natural and easily understood method of sampling - it corresponds to our intuitive notion of random selection by lot. However, simple random sampling does have its drawbacks. For instance, it may fail to provide sufficient coverage or may be impractical when the members of the population are widely scattered geographically. Next, we will examine some other commonly used sampling procedures that are often more practical than a simple random sampling.
All sampling techniques can be classified as random (probability) sampling and non-random (non-probability) sampling. Probability sampling is a sampling technique, in which the subjects of the population get an equal opportunity to be selected as a representative sample which is not the case with non-probability sampling.
Let’s assume that there are 35 desks in the classroom arranged in 5 rows with 7 desks in each row. The following diagrams illustrate the difference between random and non-random sampling of 11 students.


We discussed the simple random sampling which is the ideal sampling method for producing the least biased sample. Next, we will examine some other commonly used random and non-random sampling procedures.
Section 2: Systematic Sampling
A systematic random sampling is a method for selecting a random sample by listing the entire population and picking every k-th object starting randomly with the object numbered between 1 and k. We select k to be as close as possible to the population size divided by the sample size.
Here is an example for picking every third person from a population of size 12 starting with number 3:

Here is an example for picking every third person from a population of size 12 starting with number 2:

Here is an example for picking every second person from a population of size 10 starting with number 2.

The procedure can be outlined in the following steps:
- Divide the population size by the sample size and round the result down to the nearest whole number, k.
- Use a random-number table or a similar device to obtain a number, m, between 1 and k.
- Select for the sample those members of the population that are numbered m, m + k, m + 2k, etc.
When is the systematic sampling useful? For example, the owner of a small convenience store in a rural area wants to survey the opinion of their customers and they expect 20 customers in the next hour. They think that a sample of size 5 will be sufficient so they follow the procedure:
- The population size 20 divided by the sample size 5 is 4 so k=4.
- A random number between 1 and 4 picked by a computer is 3.
- They will survey the 3rd, 7th, 11th, 15th, and 19th customers.

There are advantages and disadvantages for every procedure. The main advantage of the systematic random sampling is that the procedure doesn’t require access to the entire population, but on the other hand it does assume the knowledge of the population size.
Section 3: Stratified Sampling
A stratified sampling is a method for selecting a random sample used to ensure that subgroups of the population are represented adequately. Here is a diagram that summarizes the stratified sampling procedure:

The procedure can be outlined in the following steps:
- Divide the population into subpopulations called strata.
- From each stratum, obtain a simple random sample of size proportional to the size of the stratum; that is, the sample size for a stratum equals the total sample size times the stratum size divided by the population size.
- Use all the members obtained in Step 2 as the sample.
When is the stratified sampling useful? It is useful when the population is heterogeneous and contains several different groups. For example, the principal of a high school wants to survey the opinions of the students. They think that a sample of size 100 will be sufficient. There are total of 1000 students (400 freshmen, 300 sophomores, 200 juniors, 100 seniors). It won’t be representative if in a sample of size 100 there were 25 students from each class. So they follow the procedure:
- The population is already divided into classes that will be used as strata.
- The sample size for each class will be determined by the formula k*n/N, where k is the class size, n is the total sample size, N is the population size.
- From each class, obtain a simple random sample of size proportional to the size of the class; that is there will be:
- Freshmen: \(200\cdot\frac{50}{500}=20\)
- Sophomores: \(150\cdot\frac{50}{500}=15\)
- Juniors: \(100\cdot\frac{50}{500}=15\)
- Seniors: \(50\cdot\frac{50}{500}=5\)
- Combine all the members obtained in Step 2 as the sample that will consist of 20 freshmen, 15 sophomores, 10 juniors, 5 seniors.
There are advantages and disadvantages for every procedure. The main advantage of the stratified random sampling is that the procedure ensures a high degree of representativeness of all the strata or layers in the population, but on the other hand it is time-consuming and tedious.
Section 4: Voluntary Response Sampling
A voluntary response sampling is a type of sampling that produces a sample made up of self-chosen participants. These participants volunteer to take part in different research studies to share their opinions on topics that interest them. Polling through call-in radio shows is an ideal voluntary response sample example. Only a part of the population that listens to the particular radio station (and who chooses to answer by dialing in) participate in the poll. The responses collected do not accurately reflect the feelings of the entire population as only those people who choose to call in and take part in the study will bother to respond. When is the voluntary response sampling useful? As long as the question doesn’t cover a controversial topic, a voluntary response sampling can be a quick and cheap way to survey the opinion of the population.
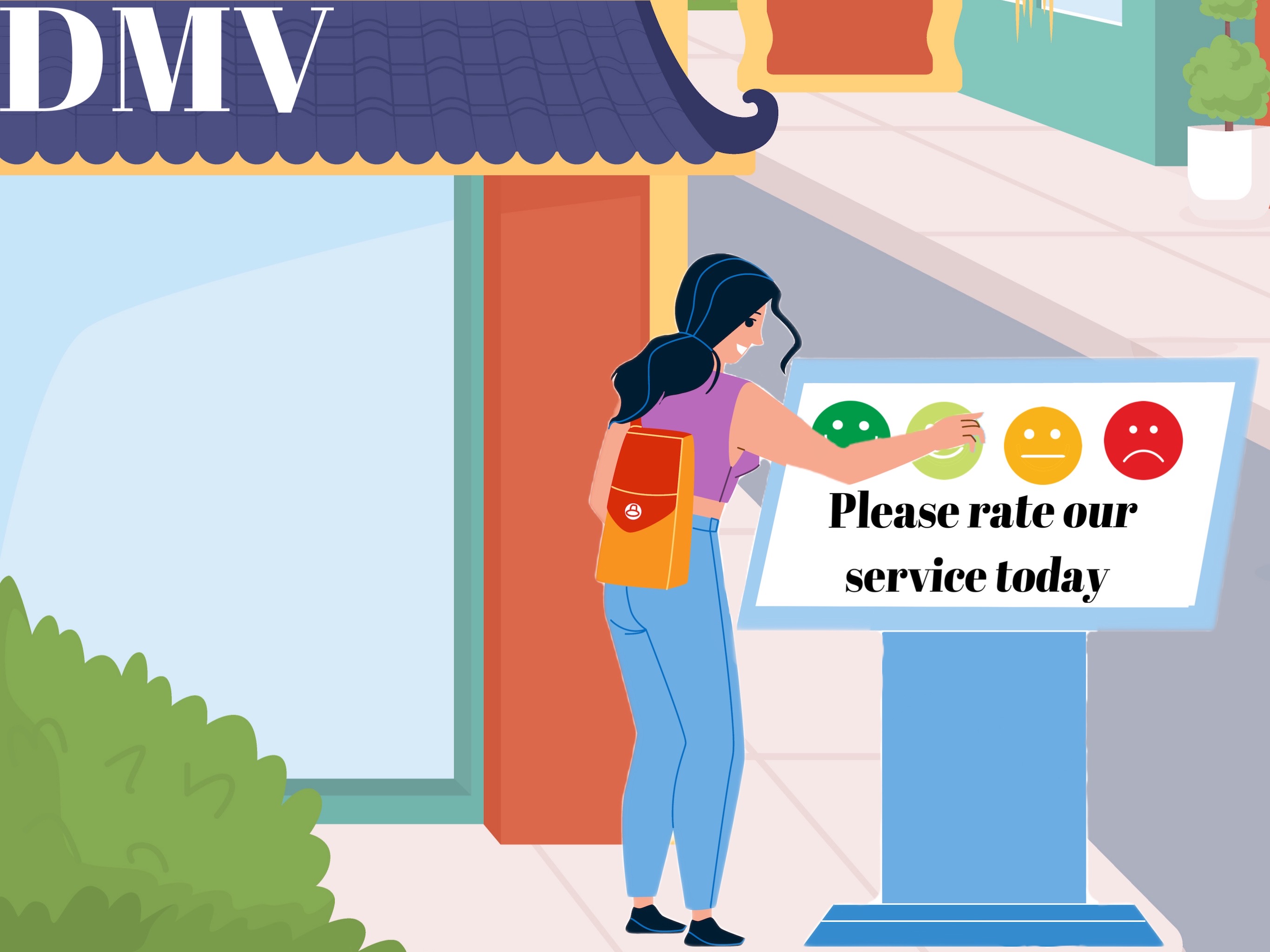
The main advantage of the voluntary response sampling is that the procedure may not accurately reflect the feelings of the entire population, but on the other hand it is inexpensive and minimal effort is required.
Section 5: Convenience Sampling
A convenience sampling is a nonrandom method of selecting a sample; this method selects individuals that are easily accessible. Here is a diagram that summarizes the convenience sampling procedure.

When is the convenience sampling useful? In pilot studies, a convenience sample is usually used because it allows the researcher to obtain basic data and trends regarding his study without the complications of using a randomized sample. The main advantage of the convenience sampling is that the procedure is convenient and inexpensive, but on the other hand it is a non-random sampling procedure, so generalization is questionable.
Section 6: Other Biases
To minimize the sampling biases, most large-scale surveys combine more than one random sampling techniques. Such multistage sampling is used frequently by pollsters and government agencies. For instance, the U.S. National Center for Health Statistics conducts surveys to obtain information on a variety of health issues. Data collection is done by a multistage probability sampling of approximately 42,000 households.
There are many other possible issues that may compromise the validity of a statistical study. Here is by no means a complete list of terms that one should be familiar with when analyzing a statistical study:
- Push polling
- Loaded questions
- The order of questions
- Social acceptability bias
- Publication bias
- Patterns in missing data
- Self-interest study
- Precise lies
Some of the terms are examples of a specific bias and some are examples of unethical practices that lead to some type of bias.
A push poll is an interactive marketing technique, most commonly employed during political campaigning, in which an individual or organization attempts to manipulate or alter prospective voters' views under the guise of conducting an opinion poll. Large numbers of voters are contacted with little effort made to actually collect and analyze voters' response data. Instead, the push poll is a form of telemarketing-based propaganda and rumor spreading, disguised as an opinion poll. Push polls may rely on insinuations, or information gathered from opposition research serving the political opponents.
A "loaded question", like a loaded gun, is a dangerous thing. A loaded question is a question with a false or questionable presupposition, and it is "loaded" with that presumption. This type of fallacious question puts the person who is being questioned in a disadvantageous and defensive position, since the assumption in the question could reflect badly on them or pressure them to answer in a way that they wouldn’t otherwise.
- One especially loaded question was asked by Brian Williams during the 2011 Republican presidential candidate debate at the Reagan Library. Directed to Texas Senator Rick Perry, Williams asked: 'have you struggled to sleep at night with the idea that any one of those [234 Texas executed inmates] might have been innocent?' This loaded question threw Perry for a loop because it actually entails a number of different assumptions including the moral validity of execution and Perry's own involvement with this part of the judiciary process.
- Here is another recent example of a loaded question: “Do you regret at all, all the lying you’ve done?” A White House correspondent on Thursday August 13th 2020 said he’s been waiting five years to ask U.S. President Donald Trump a question, and he finally got his chance. But the blunt question was not met with an answer as this is usually the best strategy in response to loaded questions such as this.
The order that questions are asked in a survey or study can influence the answers that are given. That's because the human brain has a tendency to organize information into patterns. The earlier questions — in particular, the ones that come just before — may provide information that subjects use as context in formulating their subsequent answers, or affect their thoughts, feelings, and attitudes. Pew Research gave this example from a December 2008 poll: "When people were asked 'All in all, are you satisfied or dissatisfied with the way things are going in this country today?' immediately after having been asked 'Do you approve or disapprove of the way George W. Bush is handling his job as president?'; 88 percent said they were dissatisfied, compared with only 78 percent without the context of the prior question.”
In social science research, social desirability bias is a type of response bias that describes the tendency of survey respondents to answer questions in a manner that will be viewed favorably by others.[1] It can take the form of over-reporting "good behavior" or under-reporting "bad", or undesirable behavior. The tendency poses a serious problem with conducting research with self-reports. This bias interferes with the interpretation of average tendencies as well as individual differences. When confronted with the question, "Do you use drugs/illicit substances?" the respondent may be influenced by the fact that controlled substances, including the more commonly used marijuana, are generally illegal. Respondents may feel pressured to deny any drug use or rationalize it, e.g. "I only smoke marijuana when my friends are around."
Publication bias is a type of bias that occurs in published academic research. It occurs when the outcome of an experiment or research study influences the decision whether to publish or otherwise distribute it. Publishing only results that show a significant finding disturbs the balance of findings and inserts bias in favor of positive results. Publication bias is sometimes called the file-drawer effect, or file-drawer problem. This term suggests that results not supporting the hypotheses of researchers often go no further than the researchers' file drawers, leading to a bias in published research.
Understanding the reasons why data are missing is important for handling the remaining data correctly. If values are missing completely at random, the data sample is likely still be representative of the population. But if the values are missing systematically, analysis may be biased. For example, in a study of the relation between IQ and income, if participants with an above-average IQ tend to skip the question ‘What is your salary?’, analyses that do not take into account this may falsely fail to find a positive association between IQ and salary.
Another type of bias occurs in a self-interest study – a study in which the researchers have an interest in the outcome of the study. Would you trust a 1930 Lucky Strike advertisement knowing that the medical study was sponsored by the tobacco company itself? Soon after e-cigarettes debuted in Europe in 2006, tobacco companies began investing heavily in vaping. Do you trust that vaping is harmless? I hope not!
Another interesting phenomenon called the precise lies frequently occurs in the age of mass media. The famous statistic of women earning $0.77 for every dollar men earn is exactly this sort of lie. It makes no attempt to correct for differences in experience, education, or even full versus part time status. Louis Jacobson of PolitiFact does a very nice job of walking a reader through different ways controls can be added that change that number, while also pointing out that it can be a true statement as long as it doesn’t come with an implication this difference is due to discrimination against women working equivalent jobs.
We discussed a variety of issues that may arise during the sampling process and compromise the validity of a statistical study. As a responsible citizen it is your responsibility to be aware of these issues when conducting or analyzing a statistical study.